How Kern AI uses crowd.dev
Kern AI struggled with going from developer adoption to successfully commercializing their open source AI solution. Learn in this customer story, how they tackled their problems with crowd.dev - the developer data platform unify customer, product, community, and social data.
ā 4x more pipeline generated Ā Ā ā Deals close 35% faster Ā Ā ā 8 hours saved per week
Kern AI
2x ROI or money-back
You will generate at least twice as much revenue with crowd.dev as you pay for our product in the first year. If not, you get your money back. Deal?
Kern AIās story
Kern AI is building open source developer tools for data-centric NLP. They have been in the natural language processing space for many years already - lucky enough to witness firsthand how the field has evolved in the last years. The team at Kern AI believes that the time has come to make the technology available to everyone. As language models become a commodity, they deeply believe that the true benefit for teams lies within the connection of LLMs and their data - where Kern AI comes in.
ā
Going from developer adoption to commercialization
Before implementing crowd.dev, Kern AI faced a challenge in going from developer adoption with their open source project refinery to enterprise commercialization. The problem - Kern AIās potential customers often didnāt know about the commercial offerings beyond the open source project. Additionally, Kern AI didnāt have full visibility into which developers and companies were engaging with them across channels.
This meant companies were making crucial decisions about the product long before Kern AI even had the opportunity to get in touch and, for example, present a demo. This pre-purchase buying journey meant that they had limited chances to engage, educate, and influence prospects effectively. Kern AI found it increasingly challenging to align their marketing and sales efforts, impacting their ability to showcase the enterprise value of their AI solutions.
Kern AI began to identify commercialization opportunities as early as possible. This was mainly done through intent signals from channels such as GitHub, LinkedIn, Twitter, or even product data. However, the spread of channels resulted in fragmented data and an inability to notice buying signals accurately. The lack of a centralized approach made it difficult to optimize campaigns, ensure consistent messaging, and identify new opportunities early on. On top of that, it required a lot of manual work and tab switching.
Another challenge that Kern AI faced was in identifying and persuading the economic buyers for their AI solutions. While developers were often the initial point of contact and had a strong influence on the technical aspects, they were not the ultimate decision-makers who held the purse strings. Convincing and engaging non-technical stakeholders, such as C-level executives or department heads, proved to be a complex and distinct challenge. Kern AI needed a strategy that could bridge this gap and effectively connect with the economic buyers of their offerings.
ā
How Kern AI uses crowd.dev
1. Unify community, social, product, and CRM data
Kern AI recognized the need for a centralized hub to gather, analyze, and consolidate data from various sources, including their community, social media interactions, product usage, and CRM. Through crowd.dev, they seamlessly integrated these data streams into a unified platform, providing a comprehensive view of their prospects and customers. This unified data approach allows them to gain valuable insights, understand user behavior, and identify the most promising leads, all from a single source.
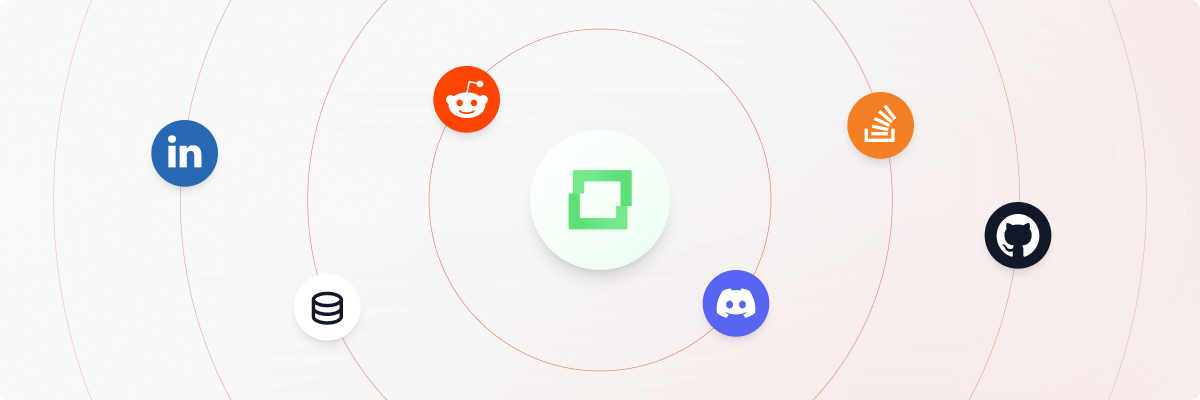
ā
2. Discover companies whose developers are already engaging with Kern AI
With crowd.dev's powerful analytics and tracking capabilities, Kern AI can pinpoint relevant companies to approach that already have one or more individual developers engaging with their product or brand. By identifying these companies, they can focus their efforts on leads with demonstrated interest, and prioritize outreach to those prospects.
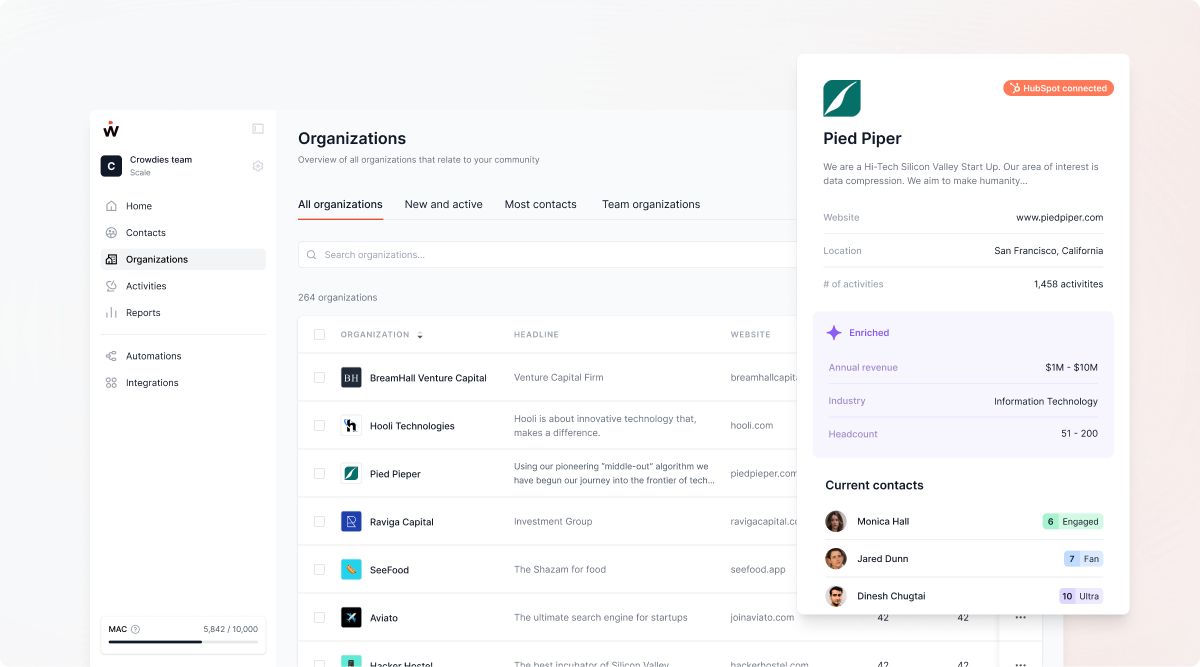
ā
3. Sync data with their CRM and build pipeline on auto-pilot
Kern AI takes advantage of crowd.dev's CRM integration. This integration ensures that the data on engaged prospects is automatically synced with their CRM, creating a pipeline that practically builds itself. This automation significantly reduces manual data entry and enhances the efficiency of their sales process. It also allows their sales team to focus on nurturing leads and converting prospects into customers.
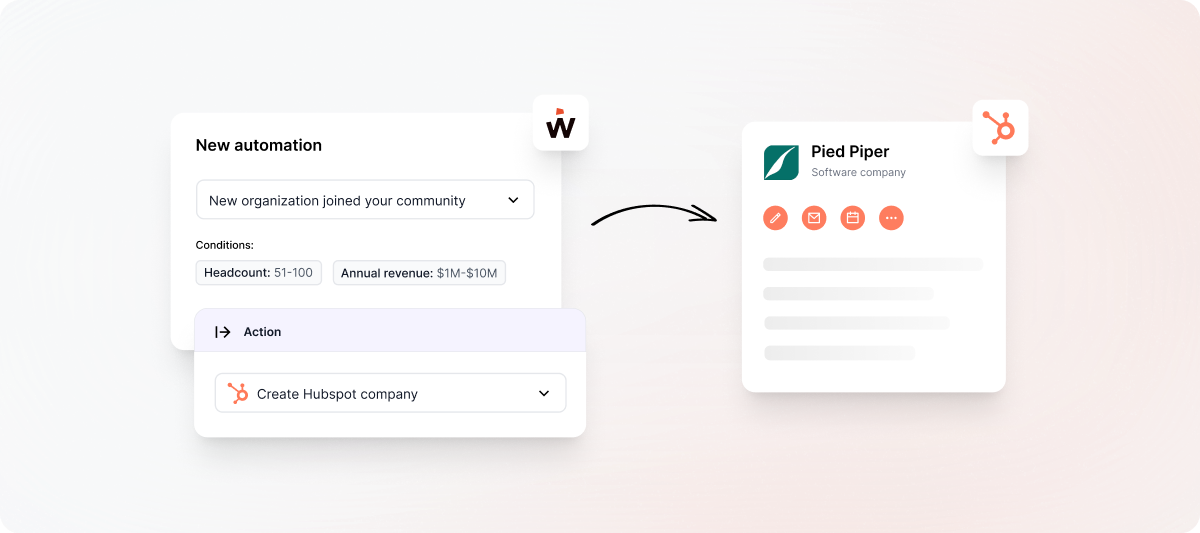
ā
4. Do warm outreach to companies that have developer buy-in
Armed with comprehensive data and a well-organized pipeline, Kern AI has adopted a proactive approach to outreach. With crowd.dev, they can engage in targeted and personalized communication with companies whose developers have already demonstrated interest in their offerings. This "warm" outreach strategy not only increases the likelihood of conversion but also enhances the overall customer experience, as Kern AI can tailor their messaging to the specific needs and preferences of each prospect - without spamming the developers in their community.
By implementing these steps with crowd.dev, Kern AI effectively overcame their previous challenges and has achieved a repeatable and efficient GTM strategy for their developer product.
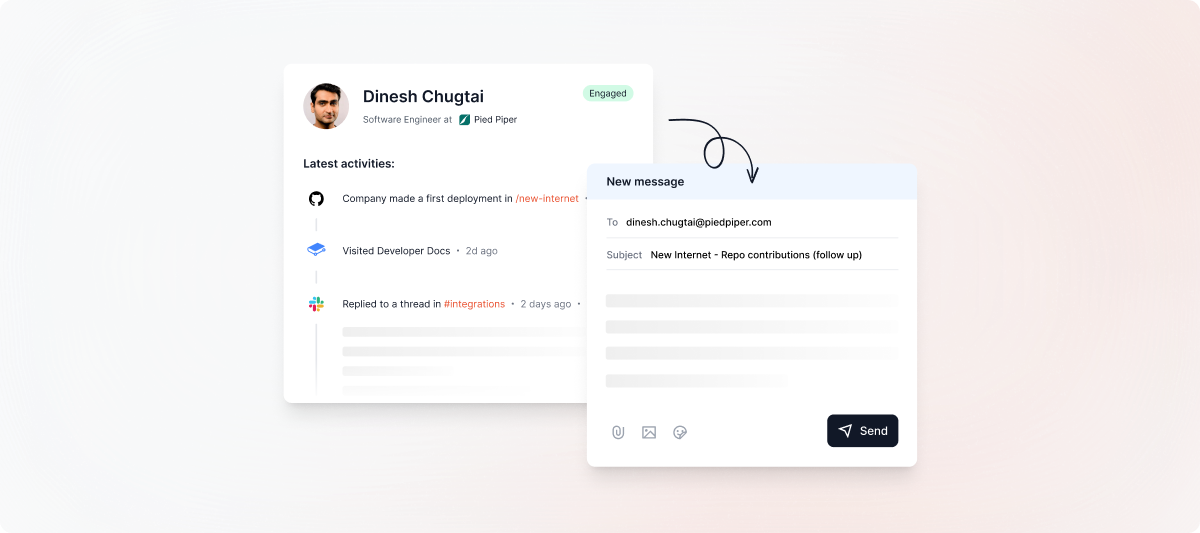
ā
Kern AI's results with crowd.dev
šø 4x more pipeline generated
ācrowd.dev uncovers companies that already use our product but havenāt engaged with our Go-To-Market team yet. This helped us to 4x the opportunities in our enterprise sales pipeline. By following an account-based approach, we can effectively reach out to decision makers without losing the trust of our open source community.ā
ā
šļø 35% faster from lead stage to closed
āWe noticed that opportunities sourced through crowd.dev closed on average 35% faster than others.ā
ā
ā³ 8 hours saved per week
āBefore, we spent countless hours trying to find a needle in a haystack. crowd.dev centralizes, cleans, and enriches all relevant data points for us. This saves us about 8 hours per week - time that we can now spend on other revenue generating activities.ā